The Evolution Journey of Conversational AI
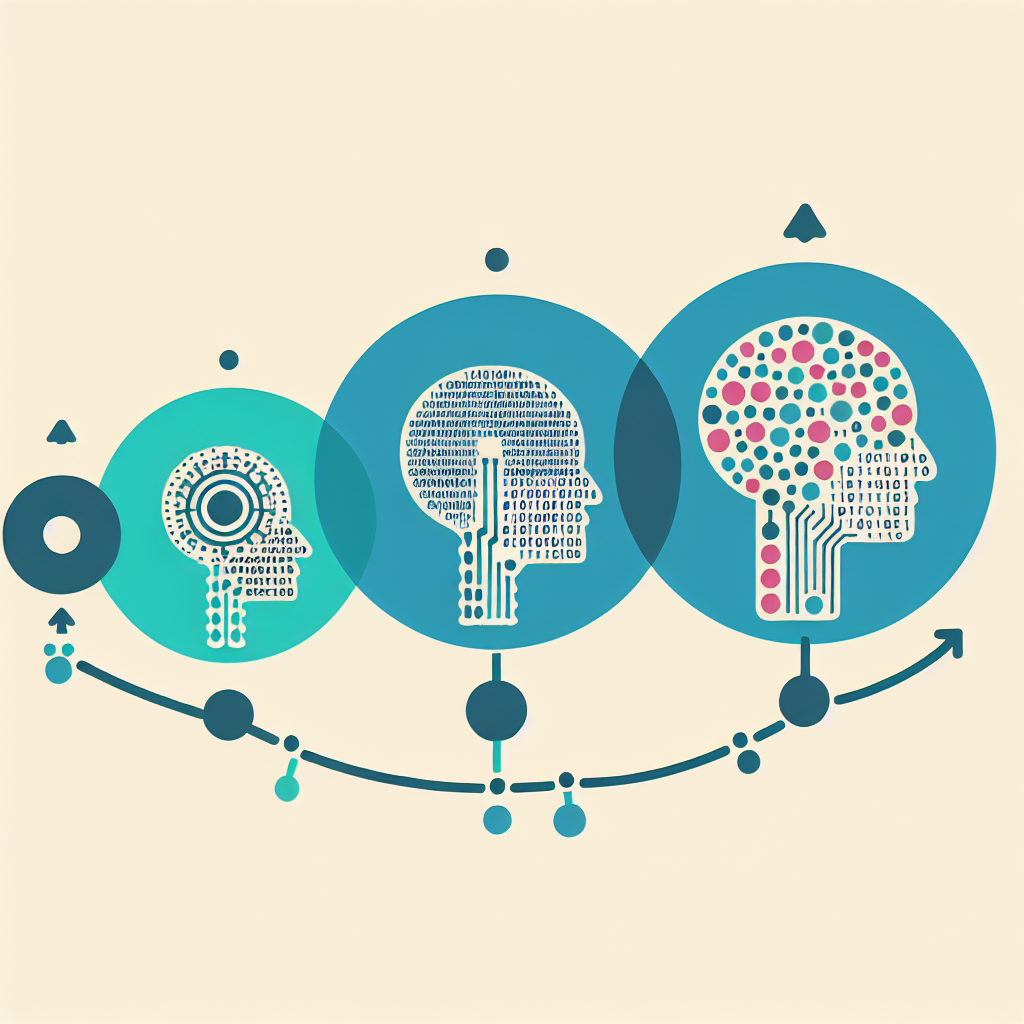
The Evolution of Conversational AI: Advancements from Rule-Based Systems to Advanced Language Models
Conversational AI has made remarkable strides since its early days, evolving from simple rule-based structures to sophisticated language models capable of intelligent and human-like interactions. This evolution is not only a testament to technological breakthroughs but also reflects our deepening comprehension of language and human interaction. Let's delve into this captivating journey.
Early Beginnings with Rule-Based Chatbots (1960s-1990s)
The initial phase of conversational AI began with rule-based chatbots, which operated based on predefined commands and pattern matching. ELIZA, created by Joseph Weizenbaum at MIT in 1966, marked a pioneering step in this field. It mimicked a Rogerian psychotherapist, responding to user inputs by recognizing keywords and delivering scripted replies. This technique created an illusion of understanding, highlighting early achievements in the field.
While significant at the time, these early systems had notable limitations. They lacked genuine comprehension and adaptability, leading to confusion with complex inputs. As a result, the dialogue often appeared shallow and mechanical.
The Shift to Statistical Models (1990s-2000s)
With advancements in natural language processing (NLP), chatbots began incorporating statistical methods, signaling a shift from rigid, rule-based systems. This development allowed for more dynamic and contextually relevant responses. For instance, statistical models enabled chatbots to predict responses based on patterns found in large text corpora, such as typical conversational exchanges.
Basic sentiment analysis was also introduced during this era, which allowed AI systems to discern the emotional tone of a user's input. However, while progress was evident, these models struggled with maintaining clarity over extended interactions and often provided responses that, though grammatically correct, missed the conversational context.
Machine Learning: A New Era (2000s-2010s)
The rise of machine learning heralded a transformative era for conversational AI. This period saw chatbots learning from data inputs, refining their interactions over time. Techniques like Support Vector Machines (SVMs) and decision trees enhanced the understanding of user intents, improving response accuracy.
IBM's Watson, although not purely conversational AI, exemplified machine learning's potential by competing in Jeopardy! in 2011. These systems, however, were best suited for narrowly defined tasks, such as customer service for specific domains, lacking broader conversational adaptability.
Deep Learning and Neural Networks Revolution (2010s)
The deep learning revolution of the 2010s significantly enhanced NLP capabilities. Neural network architectures, particularly Recurrent Neural Networks (RNNs), and later transformers, brought unprecedented advancements in language processing. RNNs, especially when integrated with Long Short-Term Memory (LSTM) frameworks, adeptly managed long-range dependencies, producing more coherent responses.
This era was marked by the advent of transformer models. BERT, introduced by Google, improved task-specific language understanding, while OpenAI's GPT demonstrated impressive text generation across various styles.
The Rise of Large Language Models (2020s)
The latest frontier in conversational AI is defined by large language models (LLMs) like GPT-3. Trained on expansive datasets, these models embody a leap forward in AI language capabilities. GPT-3, with 175 billion parameters, can perform diverse language tasks without prior task-specific training, engaging in open-ended dialogue and providing sophisticated reasoning capabilities.
The Future: Multimodal AI
Today, the focus of conversational AI research is on integrating language capabilities with other modalities. By merging vision and audio with language processing, these systems promise more contextually aware AI agents. This includes models that can describe images, translate languages, and understand auditory inputs, potentially serving as next-gen virtual assistants.
As Yepic continues to innovate in the field of real-time AI avatars and video agents, these advancements in conversational AI present exciting possibilities for more interactive and human-like digital interactions.
Please feel free to reach out to us for a demo here: team@yepic.ai